Protein-Protein Interaction (PPI)?
The collection of all interactions between the proteins of an organism is usually called the interactome .protein-rotein interactions is seen as a crucial prerequisite to understand cells function and the general principles that govern this function. Importantly, It can also lead us to better understand signal transduction and make some developmental analysis.
What is the bioinformatical method used within AtPID?
Arabidopsis thaliana Protein Interactome Database (AtPID) is an object database that integrates several prediction methods for protein-protein interaction and a wealth of information relevant to Arabidopsis thaliana biological resources. The prediction methods include the Ortholog Interactome method, the co-expression method, the SSBP GO annotation method, domain method ,the gene fusion method and the phylogenetic profiles Method .Data pertaining to thousands of protein-protein interactions, protein sublocation, protein domain information, gene expression regulation network are all extracted from the literature and related sparse datasets.
Ortholog interaction datasets
The ortholog proteins often retain similar functions, so a pair of orthologs that interacts in one organism is likely to interact in other organism5,6. Publicly available protein interaction datasets were downloaded from the DIP database (http://dip.doe-mbi.ucla.edu/dip/Download.cgi). These included the Saccharomyces cerevisiae set, rosophila melanogaster set, aenorhabditis elegans set, and Homo sapiens set. Ortholog map files were downloaded from the Inparanoid database (http://inparanoid.cgb.ki.se/). These data files provided ortholog maps between pairs of organisms. According to the conservation of ortholog interactions across the species, we transferred the information of the ortholog interaction data of other organisms to Arabidopsis thaliana, and obtained the Arabidopsis thaliana protein interaction data. If the likelihood ratio was NA, then the likelihood ratio was assigned with the maximum value available from other organisms. Ortholog Pairs were then tested against the GSP and GSN to derive likelihood ratios .
Shared biological function(SSBP GO annotation method)
Interacting proteins often function in the same biological process so proteins acting in the same process should be more likely to interact than proteins acting in distinct processes. Furthermore, proteins functioning in small, specific processes should be more likely to interact than proteins functioning in large, general processes. The following procedure was used to quantify functional similarity between two proteins: (1) identify all biological process term shared by two proteins; (2) count how many other proteins were assigned to each of the shared terms as well; (3) identify the shared biological process term with the smallest count (SSBP). In general, the smaller this count, the more specific is the biological process term, and the greater functional similarity between two proteins. Protein pairs were binned by this measure of functional similarity and then the degree of similarity was tested for its ability to predict protein-protein interactions.
Co-expression matrices
Interacting proteins often have similar gene expression patterns, so genes that are co-expressed should be more likely to interact than genes that are not co-expressed4. We collected all available AFFY Arabidopsis microarray datasets from TAIR (date up to May 2006). For each dataset, we first chose genes with values present in 50% of the profiled samples. Then Pearson correlations were calculated for each dataset and the gene pairs were grouped into 19 correlation bins (by tenths from -1 to 1, with -0.1 to 0.1 as a single bin). The degree of co-expression was then tested against the GSP and GSN to derive likelihood ratio. Finally, we selected five leaf related datasets (Submission Number is ME00319, ME00326, ME00338, ME00331 and ME00345) in which the likelihood ratios were considerably stronger and increased consistently with increasing coexpression.
Gene fusion method
The Gene Fusion Method is based on the hypothesis that pairs of monomeric proteins that are fused in other organisms tend to be functionally related or physically interacted.
Gene neighbors method
Some of the operons contained within a particular organism may be conserved across other organisms. The conservation of an operon's structure provides additional evidence that the genes within the operon are functionally coupled and are perhaps components of a protein complex or pathway. Several methods have been reported that identify conserved operons.
Phylogenetic profile method
The phylogenetic profile method uses the co-occurrence or absence of pairs of nonhomologous genes across genomes to infer functional relatedness [7,8]. The underlying assumption of this method is that pairs of nonhomologous proteins that are often present together in genomes, or absent together, are likely to have coevolved. That is, the organism is under evolutionary pressure to encode both or neither of the proteins within its genome and encoding just one of the proteins lowers its fitness. As in all of the above methods, we assume, and later confirm, that coevolved genes are likely to be members of the same pathway or complex.
Enriched domain pair
The functional units of proteins are domains, which are often repeated in various combinations in the proteins throughout the genome. It is well known that the protein interaction can be inferred from the domain interaction. We downloaded the domains from the Pfam database (http://www.sanger.ac.uk/Software/Pfam), and then searched domains in GSP protein pairs. The protein pairs containing these domains are considered to interact with each other. In this way, 5,337 pairs involving 438 proteins were found in genome-scale. Domain pairs were then tested against the GSP and GSN to derive likelihood ratios
Bayesian Network Approach
The Bayesian Networks approach was used to integrate the six predictive data sources and build a model to predict novel protein-protein interactions. A similar approach was applied to predict yeast protein complexes and was applied here as described by Jansen, 2003. The essence of the approach is to provide a mathematical rule explaining how to adjust the odds that a pair of proteins interacts given some predictive evidence. The prior odds of interaction were defined as:
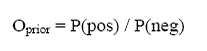
Where P(pos) is the probability of finding an interacting pair of proteins among all pairs of proteins, and P(neg) is the probability of finding a non-interacting pair. The posterior odds or the odds that two proteins interact given new predictive evidence were defined as:

Where fi is a protein pair' value in dataset i. The likelihood ratio(LR show as the equation below):
Relates the prior odds and the posterior odds as defined by a derivation of Bayes rule:

When the evidence types integrated are independent (or non-redundant), the likelihood ratio can be calculated simply as the product of individual likelihood ratios from the respective evidence types. This is known as a Naive Bayes Network:
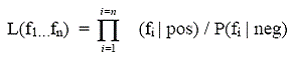
Individual likelihood ratios are easily calculated by counting the number of protein pairs with particular values (or bins of values) in the predictive dataset that overlap with the GSP and GSN sets. Also, the naive Bayes network is desirable because it lessens the initial data requirements and computational complexity required to build the model.
Intuitively, we anticipated that the majority of the data sources integrated would be nonredundant as the methods used to generate the data are unrelated thus the source for false positive predictions should be unrelated. After reviewing the evidence sources, we found that the shared biological function and enriched domain pair types were in some cases redundant, for example, where proteins are assigned to a biological processes based on their domains. So, to guard against over-estimating likelihood ratios when combining these evidence sources, we made an exception to the na茂ve Bayes model, and combined these two sources to a full Bayes model, that is we generated likelihood ratios for protein pairs binned by their values in both evidence sources. (See Redundant Data section above)
What is GSP and GSN?
Gold Standard Positive Interactions (GSP): Arabidopsis thaliana GSP is the protein pairs which have real interactions confirmed before retrieved from Pubmed, KEGG, and IntAct Database. GSP is used to compute LR score. We collected GSP manually from literature, the complex type interaction in GSP were collected from KEGG and the GSP pairs in IntAct DB were also added into the GSP datasets.
Gold Standard Negative Interactions (GSN):
The gold standard negative interaction set (GSN) was defined as all protein pairs in which one protein was assigned the plasma membrane cellular component and the other the nuclear cellular component, as assigned by Gene Ontology Consortium. The pairs in GSN means Protein ineraction won't occur in cell. They are used by the Bayesian Network as the negative datasets. We also used GSN to compute the LR score.
What does the displayed number mean when your mouse moved over a certain icon?(LR)
LR -the likelihood ratio which is calculated as Pr(CL|GSP) / Pr(CL|GSN), is directly related to the likelihood that two proteins interact. Through our integration method with Bayesian, High LR assigned to each predicted protein-protein pair indicates the possible of the interaction between the two proteins. The higher the LR is, the more possible the interaction relationship occurs between them.We have computed out LR cut-off to filter raw prediction results. The LR cut-off is 217.
GSP/GSN -the number of protein pairs in given classes that were present in the gold standard positive/negative set of interactions.
Pr(CL|GSP or GSN) -the probability of a protein pair having being part of a class given that it was present in the GSP/GSN.
The possible counts are the number of GSP/GSN interactions between two proteins represented in the respective datasets.
e.g. Ortholog interaction datasets
Caenorhabditis elegans (CE)
